The minsadbesd approach
Consider the model composed by
observations
which are put in the forms
,
where
,
,
,
are real functions defined on closed interval
(see Goetschel&Voxman, Ming,
Friedman and Kandel). The model
is approximately described by a regression line given by the equation
,
of form
,
where
const.
,
const.
.
Thus we have the initial relation
.
For the inputs
the distance between an observed value
and the corresponding theoretical value
is:
if

and
if
.
Case 1:
.
In this case we solve the problem under the assumption that
.
The minsadbesd algorithm lead us to solve the problem
(1.1)
or

(1.2)
For all
,
,
we make the substitutions:
,
,
,
.
Thus (1.2) is equivalent to


(1.3)
or
(1.4)
where
,
,
.
Let
.
For function
,
we have
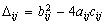
The sign of the discriminant is unknown. We have four cases which depends on signs
of
;
consequently, the graph of
has one of the forms shown in Fig. 1-4.
Case 1.1.
The "easy" case appears when all the discriminants are negative, namely
.
In this situation the functions have the forms shown in Fig. 3 or Fig. 4.
The problem (1.4) is equivalent to
(1.5)
where
( this writing means that some of the terms are positively and the others
are negatively, depending on the concrete signs of


The unique minimizing point for the function
(see also Fig. 5) is

Case 1.2.
have random signs.
The graph of the continuous function
is composed from small pieces which are parts from the functions given by the equations

are real numbers with general form


(see Fig. 6). We consider the following sets:



Thus the feasible set is

which is relatively easy to settle, as in Section 2.
Case 2:
.
(1.6)
gives

(1.7)
The problem (1.7) is equivalent with


(1.8)
which becomes
(1.9)
if
.
We denote
.
For function
,
we have
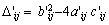
Case 2.1.
First, we consider the case
.
Thus the graph of
has one of the two forms shown in Fig. 3 and Fig. 4.
Then the problem (1.9) is equivalent with
(1.10)
where


The unique minimizing point for function
is
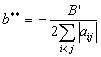
The approach is the same as in first case but with other coefficients.
In both situation,
is obtained from the condition that the line pass through the initial fixed point.
Case 2.2.
All the comments stored in case 1.2 keeps their validity.
For


we have

and (1.4) is equivalent with
(1.11)
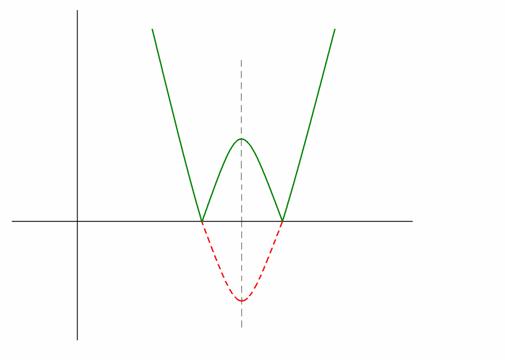
Figure 1. The graph of
(green) if
and

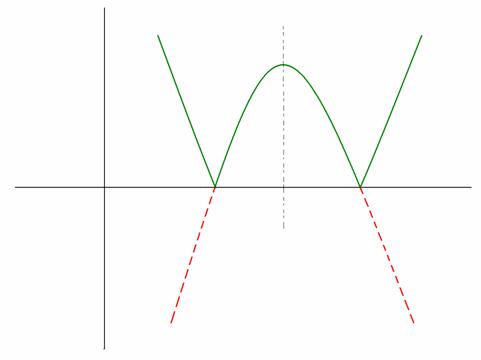
Figure 2. The graph of
(green) if
and

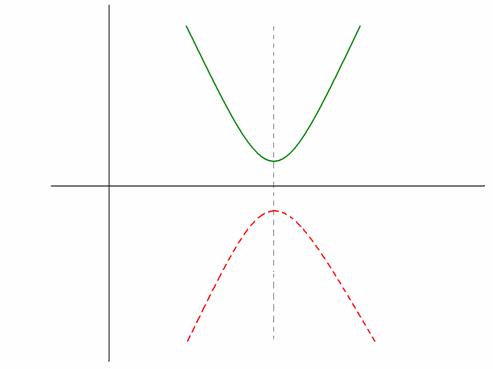
Figure 3. The graph of
if
and

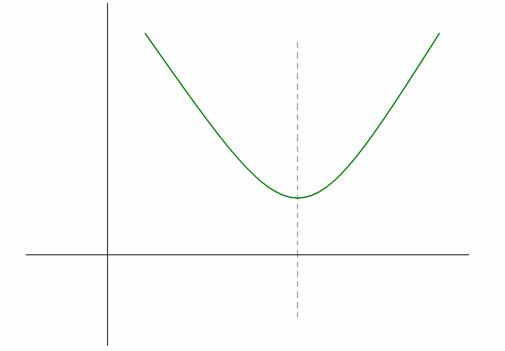
Figure 4. The graph of
if
and

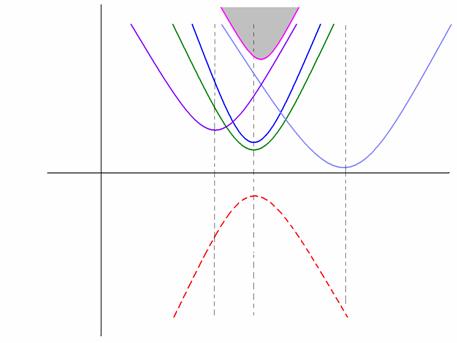
Figure 5. The graphs of the functions
,
when
;
the surface bounded by the graph of
is colored in gray
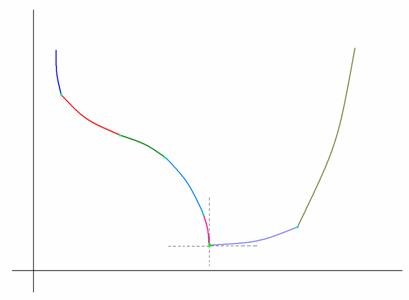
Figure 6. The graph of the function
when
have random signs
Accordingly to the facts proved in the preceding chapters, namely Section 1, case
1.2, the set of feasible points is
( notice: for this example we obtain



References
1. Arthanary T. S., Yadolah Dodge, Mathematical Programming in
Statistics, John Wiley and Sons,
New
York
, 1980
2. Wu Cong-Xin and Ma Ming: Embedding problem of fuzzy number
space: Part I, Fuzzy Sets and Systems 44, 1991, p. 33-38
3. Wu Cong-Xin and Ma Ming: Embedding problem of fuzzy number
space: Part III, Fuzzy Sets and Systems 46, 1992, p. 281-286
4. P. Diamond and P. Kloeden, Metric spaces of fuzzy sets,
Fuzzy Sets and Systems 35, 1990, p. 241-249
5. P. Diamond, Fuzzy least squares, Inform. Sci. 46,
1988, p. 141-157
6. P. Diamond and P. Kloeden, Metric spaces of fuzzy sets, Corrigendum,
Fuzzy Sets and Systems 45, 1992, p. 123
7. R. Goetschel, W. Voxman, Elementary Calculus, Fuzzy
Sets and Systems 18, 1986, p. 31-43
8. I.M. Hammerbacher and R. R. Yager, Predicting television revenues
using fuzzy subsets, TIMS Stud. Management Sci. 20, 1984, p. 469-477
9. A. Katsaras and D. B. Liu, Fuzzy vector spaces and fuzzy topological
vector spaces, J. Math. Anal. Appl. 58, 1977, p. 135-146
10. Ma Ming, M. Friedman, A. Kandel, General fuzzy least squares, Fuzzy
Sets and Systems 88, 1997, p. 107-118
11. C. V. Negoita and D. A. Ralescu, Applications of Fuzzy Sets to Systems
Analysis, Wiley,
New York
, 1975
12. H. Prade, Operations research with fuzzy data, in: P. P. Wang and
S. K. Chang, Eds., "Fuzzy sets: Theory and Application to Policy Analysis and Information
Systems", plenum,
New
York
, 1980, p. 115-169
13. M. L. Puri and D. A. Ralescu, Differentials for fuzzy functions,
J. Math. Anal. Appl. 91, 1983, p. 552-558
14. H. Tanaka, H. Isibuchi and
S. Yoshikawa
, Exponential possibility regression analysis, Fuzzy Sets and Systems 69,
1995, p. 305-318
15. H. Tanaka,
S. Uejima
and K. Asai, Linear regression analysis with fuzzy model, IEEE Trans. Systems
Man Cybernet SMC-12, 1982, p. 903-907
16. H. J. Zimmermann, Fuzzy programming and linear programming with several
objective functions, Fuzzy Sets and Systems 1, 1978, p. 45-55
17. R. R. Yager, Fuzzy prediction based upon regression models, Inform.
Sci. 26, 1982, p. 45-63